Natural Language Processing Applications in Content Marketing
Natural language processing, artificial intelligence and machine learning; these are all terms you’ve probably heard in content marketing conversations recently. It’s all part of a growing trend to automate content research, creation and tracking, allowing marketing teams to create content at scale.
But are there truly natural language processing applications in content marketing? Can this still-maturing tech analyze and create quality content that is valuable to your users?
The simple answer is… we’re getting there. And with each passing year, the capabilities of artificial intelligence (AI) grow stronger and more varied.
In the past, AI and machine learning have gotten a bad rap in content marketing circles. Processes like machine translation and natural language generation have been dressed down for their clunky, and sometimes incoherent, results.
But the AI field has expanded and developers have fine-tuned their processes, creating programs that marry big data processing capabilities with traits of the human brain. The use of AI by content marketers has become much more feasible.
And anything that can streamline your processes and help you with content creation at scale is a welcome change.
Let’s see how some of the technology in AI and natural language processing can help you now, and in the future.
Machine Translation
Machine translation is exactly what it sounds like. It’s the process through which computers translate content from one human language to another without the help of humans.
In the beginning, translation software and apps like Google Translate could only translate at the word or phrase level, using rules-based programming. That means they could only translate using only preprogrammed language rules.
And the translations were quite literal.
Now, however, apps can translate whole texts at the semantic level. Developers have figured out how to create multi-layered neural networks that work a lot like our own brains. It’s a type of machine learning that actually learns both the literal and semantic meaning of text to produce a translation that actually makes sense to the language speaker.
Machine translation is a great tool for content marketers working in global markets where their language is not the first one spoken. It even applies to content created for one country, where many languages are spoken.
In the United States, for example, there are an estimated 41 million people who speak Spanish at home. Imagine if you could translate your English-language content for the Hispanic market and do it at scale.
Still, apps like those from Google and Facebook get a lot of flack for their awkward translations, particularly for more complex language structures. I often use the translate functions on Google or Facebook to translate posts from my Israeli friends from Hebrew to English. The results are less than stellar.
It also leads to some interesting situations, as can be seen in the SERP for the search term “beignes.” This French term for the word “donut” returns a number of results in French because my search setting is set to Canada.
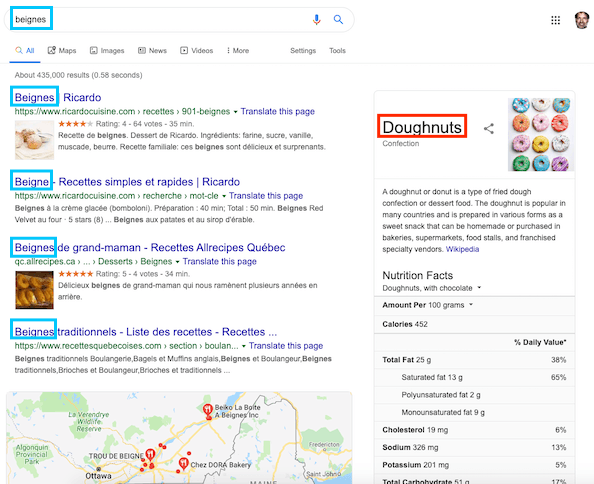
Yet Google’s Knowledge Graph is in English.
And that’s where you have to be careful. Although the algorithms are sophisticated, they lack true natural language understanding, that seems to be innate to human beings.
Machine translation tools can’t put content into cultural context. Slang, idioms and cultural references are literally lost in translation, and some translations can be downright offensive.
Before translating anything for another market, it’s important to understand if the topic and cultural references will, at best, simply not resonate with the target audience or, at worst, offend your audience outright. And avoid using idioms specific to your country or culture.
Speech Recognition
Speech recognition has turned out to be very useful for content marketers. MarketMuse has turned a number of their webinars into blog posts featuring video and text that’s been automatically transcribed from the video.
There are a number of real-world applications that can do this efficiently and cost-effectively. Wistia, which we use for video hosting, offers transcription services that’s built into their workflow, which is much appreciated.
Like other automated transcription services, they correctly process about 80% of the translation from audio to text. However, the remaining 20% still needs to be done by a human.
In the case of the MarketMuse blog, you won’t see that text in the blog post. Instead, it’s incorporated as structured data.
Sentiment Analysis
Sentiment analysis uses machine learning and natural language processing to understand the negative or positive connotation behind a text. Some organizations, like IBM, refer to it as tone analysis. They analyze the emotions and tones exhibited in online content to predict whether that person is happy, sad, and more.
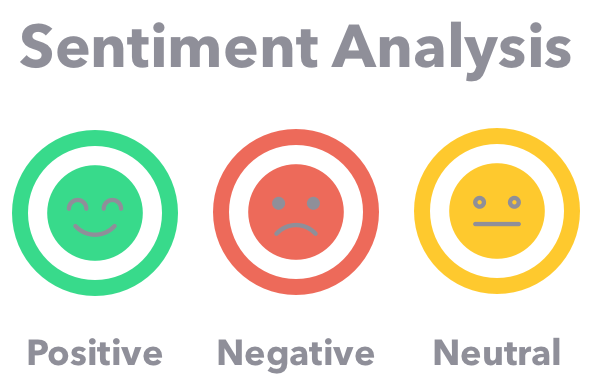
It’s very useful for social listening and A/B testing social media posts. A sentiment analysis tool can read social media posts looking for positive or negative words to classify it.
According to CMSWire, The sentiment behind some posts is pretty easy to figure out:
I love this new hair product! (Positive)
Plastic sandwich bags are terrible for the environment! (Negative)
Here are the latest jobs numbers from the U.S. Department of Labor. (Neutral)
The tricky part of sentiment analysis is when posts are taken out of context and they don’t have any obvious indicators of sentiment, words such as like and terrible. Without those flags, a basic analysis tool has trouble determining sentiment.
And then there’s sarcasm. I can’t speak for the rest of the world, but in the U.S. sarcasm is ingrained in our cultural rhetoric.
If you post something like “Oh great, another hurricane.” A computer running a rules-based sentiment analyzer might actually tag it as positive. We humans know, of course, that you really don’t mean it that way.
So, developers have turned to the same neural network processing they use for translation software to understand semantic meaning and learn from previous analyses.
It’s a great tool for measuring how your audience is reacting to your posts. But marketers have actually started using it in another way.
They’ve started to turn sentiment analysis back on their own content. Now, they’re using it to predict the emotional reaction users will have to their blog posts, social posts or even their web copy, before they release it.
By using sentiment analysis, they can A/B test anything they produce to ensure their content is having the most meaningful impact on their target audience.
Automatic Summarization
There are entire companies that make their money by summarizing and abstracting content. Rows and rows of human workers read volumes of text to distill it into key points and main ideas.
But what if a machine could take care of that? With automatic summarization, it can.
This is where, again, NLP and machine learning come in. Apps and programs use natural language processing to understand large amounts of text and pare it down to the most essential ideas.
There are two main approaches to text summarization. Extractive summarization selects the most important sentences in an article and arranges them in a meaningful manner. Each extracted sentence is preserved without any modification.
Abstractive summarization takes it a step further. Instead of using those important sentences verbatim, it paraphrases the contents.
You might wonder how that applies to content marketing. After all, aside from perhaps summarizing a blog post for a landing page, when do you have to distill your content into just a few points?
Automatic summarization can actually streamline a number of tasks to help you create and disseminate content at scale.
First, you can use content abstracts for blog posts or white papers to populate your newsletter or create posts on social media.
How about automatically creating excerpts for use in your CMS and meta description? Google already writes its own meta description if your page doesn’t have one, or isn’t deemed appropriate for the search term.
I’ve tried out some of the free text summarization apps currently available, but the results are less than spectacular.
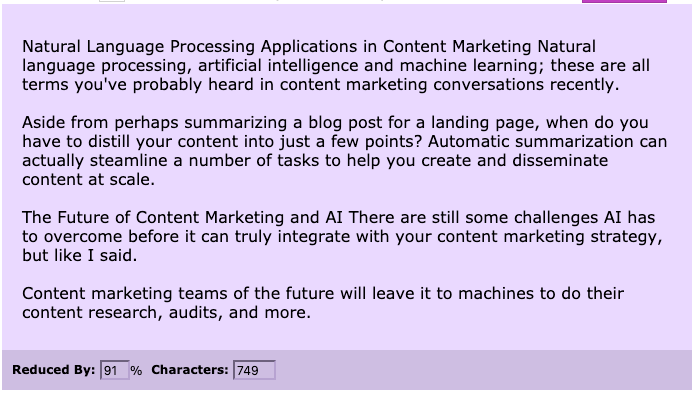
Automatic summarization also can potentially help you index content or pull keywords from your summaries to tag and index content for future audits, as well as for search.
In addition, this technology could dramatically affect the way content is curated. Even with the help of curation software such as industry-leading Curata, content curation involves a significant amount of manual effort.
Pages are still read by humans who then summarize its contents. But, natural language processing has the ability to automate and augment this process, in the same way MarketMuse does for content creation.
Finally, you can turn automatic summarization around and use it on competitors or other media outlets to conduct competitive and content research.
Personalization
The combination of machine translation, speech recognition, sentiment analysis, and automatic summarization can potentially bring a greater degree of personalization to the content experience.
Social media trends, and those within published content can be rapidly identified with content being quickly added to further capitalize on the heightened interest.
The Future of Content Marketing and AI
There are still some challenges AI has to overcome before it can truly integrate with your content marketing strategy, but like I said. We’re getting there.
With all that is available — natural language processing, understanding and generation; automatic summarization; machine translation; deep learning; and sentiment analysis — there is opportunity for content teams to automate and remove rote tasks from writers and editors.
Content marketing teams of the future will leave it to machines to do their content research, audits, and more. In fact, MarketMuse does this while providing you with some amazing insights into your content.
They will create basic, templated content, populate their newsletters, tag and index their existing content, and track what they post not only for analytics but for emotional impact, as well.
AI will free up content creators to focus on more complex, in-depth content, the kind that only a human brain can produce.
What you should do now
When you’re ready… here are 3 ways we can help you publish better content, faster:
- Book time with MarketMuse Schedule a live demo with one of our strategists to see how MarketMuse can help your team reach their content goals.
- If you’d like to learn how to create better content faster, visit our blog. It’s full of resources to help scale content.
- If you know another marketer who’d enjoy reading this page, share it with them via email, LinkedIn, Twitter, or Facebook.
Laurie is a freelance writer, editor, and content consultant and adjunct professor at Fisher College. Her work includes the development and execution of content strategies for B2B and B2C companies, including marketing and audience research, content calendar creation, hiring and managing writers and editors, and SEO optimization. You can connect with her on Twitter or LinkedIn.