Keyword Relevance: Here’s the Secret SEO Metric You Need to Know
The secret to search engine optimization (SEO) and information retrieval is to create “high quality content” that shows “topical authority,” which relies on topical relevance.
The trouble with words like quality content and topical authority is that they can be hard to measure. Certainly, you can look at your analytics to see how many read your post and for how long. Indeed, technical marketers continue to spend their day knee-deep in the minutiae of their site’s data. They’re still busy analyzing conversions, optimal keywords, volume, and cost per click. In which case, they have trouble seeing the forest for the trees.
But that doesn’t help you when you are planning your content strategy or are in the midst of your creation process. It may be time for you to take a step back. Look at your overall content and ask yourself two questions.
- Does my content do a good job of covering all relevant topics ?
- Does it signal to Google that I am an authority in my industry?
To answer these questions, we have to separate the terms “keyword relevance” from “topical relevance”.
In this article, we are going to look at the differences between the two, how they work together, and how you can use MarketMuse to score your relevance – before and during the creation process.
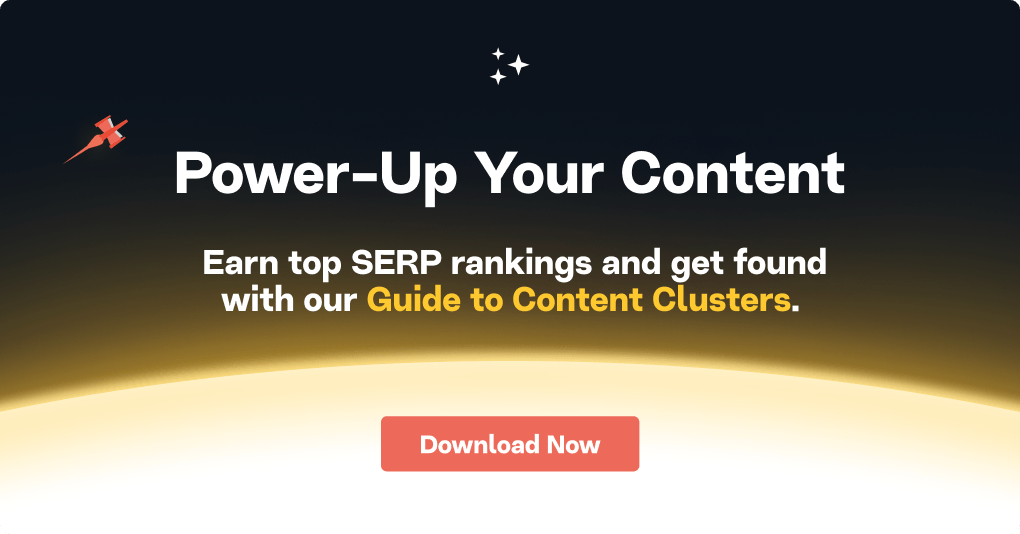
Keyword Relevance vs Topical Relevance
One school of SEO thought has been to identify relevant keywords and create dedicated pages for each. Identifying a relevant keyword stems to when people searched using only one or two words to find what they were looking for. In measuring keyword relevance, we would see how many times the word was used on the page. That was a good strategy, but it isn’t enough.
Unfortunately, search engine marketing (SEM) still operates by buying keywords –and as such, product marketers and marketing executives errantly think in terms of a single word and a corresponding page – and that’s it.
But if you want to rank for a keyword, you need to have many pages that mention that term and its related topics. In fact, Google actually looks at the topical relevance of your site – in relationship to the keywords you are targeting. Google’s charter is to offer the best possible user experience, and it does so by matching the most relevant content to a query. To do so it needs to understand the search intent.
Topical relevance isn’t just about choosing terms that are applicable to your business or service. It’s about using all the semantically relevant terms and topics in your content on your site.
Authoritative content should dive into the nitty-gritty – so you can establish that you are the expert on the subject matter. Search engines use crawlers to determine relevance, so internal linking between topically-related pages is critical. It’s a way to feature your keywords and establish topical relevance.
Why Topic Clusters Are Important
Let’s dig a little deeper into topical relevance. As noted, Google’s search algorithms look beyond keyword use to gauge the semantic meaning behind content. It does so by analyzing the path that people take from search query to landing on the right content.
As such, having comprehensive topic coverage and relevant content sends both direct and indirect signals to the search engine.
As Eric Enge explains in the video below, Google’s view of your keyword relevance matters.
“It’s often not enough just to build a single great page with the “right answer.” You have to show Google that your site is relevant for the topic in which you want to rank.”
Eric Enge
Right, so how do we identify those chunky middle terms and related topics? Next, we’ll talk about what Google does – and doesn’t – tell you, and how to work around these gaps in knowledge.
Search Engines and Keyword Relevancy: What We Know and What We Don’t
Industry experts, and Google itself, urge marketers to write high-quality content. Focus on your target audience. Which topics are relevant to them?
Google is certainly calculating relevance and scoring it in a quantitative manner. These relevance calculations are core to determining Topical Authority. Unfortunately Google doesn’t provide clear direction on how to model relevance. So marketers have found workarounds when developing content strategies. As SEMRush notes, it’s important to use topic clusters, and create valuable content that is thematically linked – as well as physically linked. An internal link between pieces of related content is a means of showing your authority on the subject matter. As you’d expect, however, it’s difficult to create topically relevant content at scale. Marketers have to manually build a topic cluster for each topic that they’re marketing, by persona, and for each part of the journey. The process can quickly get out of hand. This is one of the main reasons we built MarketMuse.
How to Get Relevant Topics and Keywords in Your Content
SEO tools today also fail to provide relevance-based metrics. Great keyword research tools such as SEMRush and SpyFu return keywords that have some degree of relevance but they can’t quantify the degree of relevance, so their data can only be sorted on Search Volume.
But there is another issue regarding the way that relevant keywords are generated. The easiest way for a keyword tool to generate suggestions is to look at the seed term you’ve entered (e.g. “canned dog food”). Then expand it to a list of niche keywords that contain the seed (e.g. “best canned dog food for senior dogs”). Campaigns relying on long-tail keywords have always relied on this type of expansion.
However, with Google’s move towards semantic search, it’s made adjustments to the way it lists Search Engine Result Pages (SERPs). Google is now lumping results, meaning that it shows the same SERP for many long-tail keywords. As a result, you won’t necessarily have a higher ranking for “canned dog food” and “best canned dog food for senior dogs.”
MarketMuse bridges this gap and provides marketers the relevant data that they need to be effective. Working with a team of Ph.D. statisticians, we’ve built the MarketMuse Keyword Relevance Engine. It’s the core technology that powers our set of content analysis tools. MarketMuse patented systems and methods for semantic keyword analysis differ from any other keyword research tool in two important ways:
- MarketMuse generates topically related keywords that don’t necessarily contain the seed term. Using our technology to research the topic ‘how to grow avocados’ returns topics including ‘watering,’ ‘fertilizer,’ ‘sunlight,’ and ‘root rot’ because they are semantically related to that subject.
- For each related keyword, MarketMuse calculates a Relevance score, which measures the degree of topical relevance. We return a list of 50 semantically related topics, sorted by relevance, so you know the most important topics upon which to focus.
In addition, we also provide the suggested distribution and variants for each topic. The suggested distribution indicates how frequently an expert mentions the topic when discussing the main subject.
Again, we’re not talking about keyword density; that’s not a thing anymore. (Keyword stuffing will actually have a negative effect.) Instead, what the suggested distribution does is provide context for how to naturally incorporate topics. Keep in mind that a topic can be important even if it’s mentioned infrequently.
Let’s say we want to create content around the topic “how to grow an avocado.” We can see from the chart below that a related search term might be about “root rot.” The more of these types of tangential topics you create around your topic cluster, the more authoritative your site becomes.
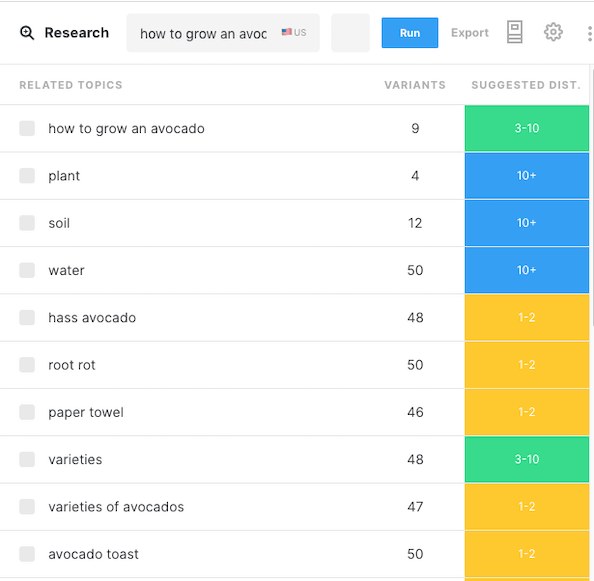
Topic variants around that phrase would include, ‘treat root rot.” Variants can be helpful in adding variety to your writing to further engage your audience.
Semantic Relevance Content Score
The beauty of MarketMuse is that in addition to all this research to help you create your content briefs, you can also optimize your content for semantic relevance.
In the graphic below, I wanted to optimize this article for “keyword relevance.” My semantic relevance score was 37, while the target was 35. So I can see that my content is ranking appropriately.
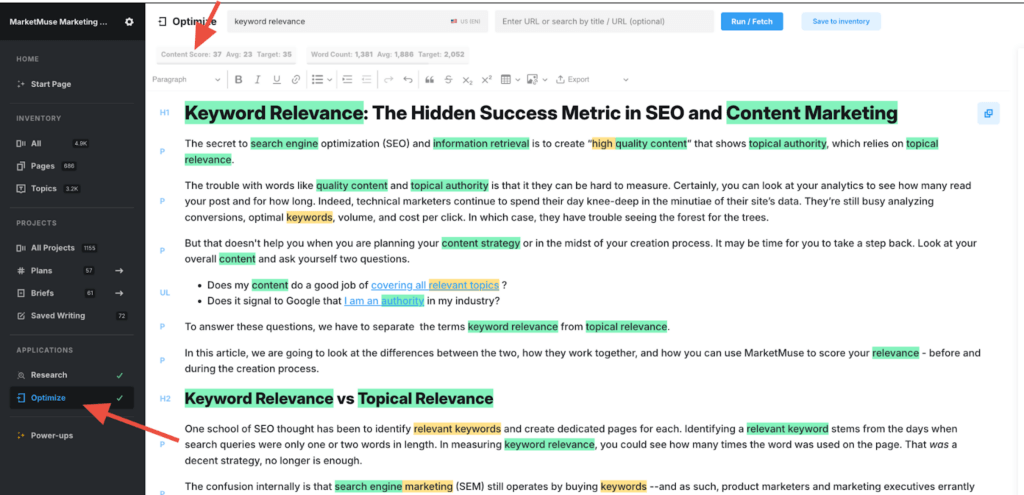
The ability to see what’s necessary to win a topic – and adjust accordingly, is invaluable.
When you create content that draws a relevant audience, that content is much more likely to drive real results to your bottom line. A central question in organic search marketing is conversion: you draw pageviews on your website, but how many visitors will convert to customers? By prioritizing relevance, you’re more likely to draw the right audience that will actually buy your products and services.
What you should do now
When you’re ready… here are 3 ways we can help you publish better content, faster:
- Book time with MarketMuse Schedule a live demo with one of our strategists to see how MarketMuse can help your team reach their content goals.
- If you’d like to learn how to create better content faster, visit our blog. It’s full of resources to help scale content.
- If you know another marketer who’d enjoy reading this page, share it with them via email, LinkedIn, Twitter, or Facebook.