7 Best Practices for Creating Content Using Large Language Models
Whether you use ChatGPT or something else, creating content with large language models (LLMs) can be a daunting task. But with the right strategies in place, it can be easier.
Setting a clear aim and understanding the model’s limitations are key steps, as are choosing a hybrid strategy and monitoring quality standards. But that’s not all. You need to provide context and directions, refine output gradually, plus verify and cross-check any facts. They’re all critical steps in the process.
1. Set a Clear Aim and Intention
Having an established aim from the start provides focus, applicability, and uniformity in your interactions with a large language model. You can customize the prompts, questions, or limits you give the model to get the preferred output. This customization boosts the applicability and quality of the created content. Establishing a determined goal simplifies evaluating and assessing the success of whatever you create.
2. Understand the Model’s Limitations
Grasping a large language model’s weaknesses is a vital step in content formation. Doing this allows you to make use of the language model more efficiently, reduce its handicaps, and raise the total quality and dependability of the generated content. It helps set realistic expectations, find errors, interpret material in context, enhance prompts, apply ethical judgment, and advance the model step by step.
3. Choose a Hybrid Strategy
A hybrid strategy to content production combines the capabilities of large language models with human management and involvement. Time and resource efficiency are the biggest elements in using large language models. But keeping humans in the loop provides quality control, contextual understanding, and the fine-tuning of answers.
Plus, humans are better prepared to handle ethical considerations, and incorporate creativity and originality. Ultimately, humans are responsible for using the output of an LLM, so it makes sense that they have a major role.
There are a couple of ways to do this:
- Let the LLM do it’s thing and spend time generating and fine-tuning your prompt. Then edit the the output to reflect your company’s norms and standards. In this scenario, an editor also must be a subject matter expert, which can be a challenging position to fill. Plus, the output may be of such poor quality that you’re better off starting from scratch as opposed to editing yourself out of that mess.
- Use the LLM interactively during the content creation process. Think of it as a sophisticated writer’s assistant that can help create outlines, expand concepts, and summarize multiple points into one cohesive whole. In this case, the writer has an expanded role. But it’s one that should be easier to fill than finding subject matter experts that are also great editors.
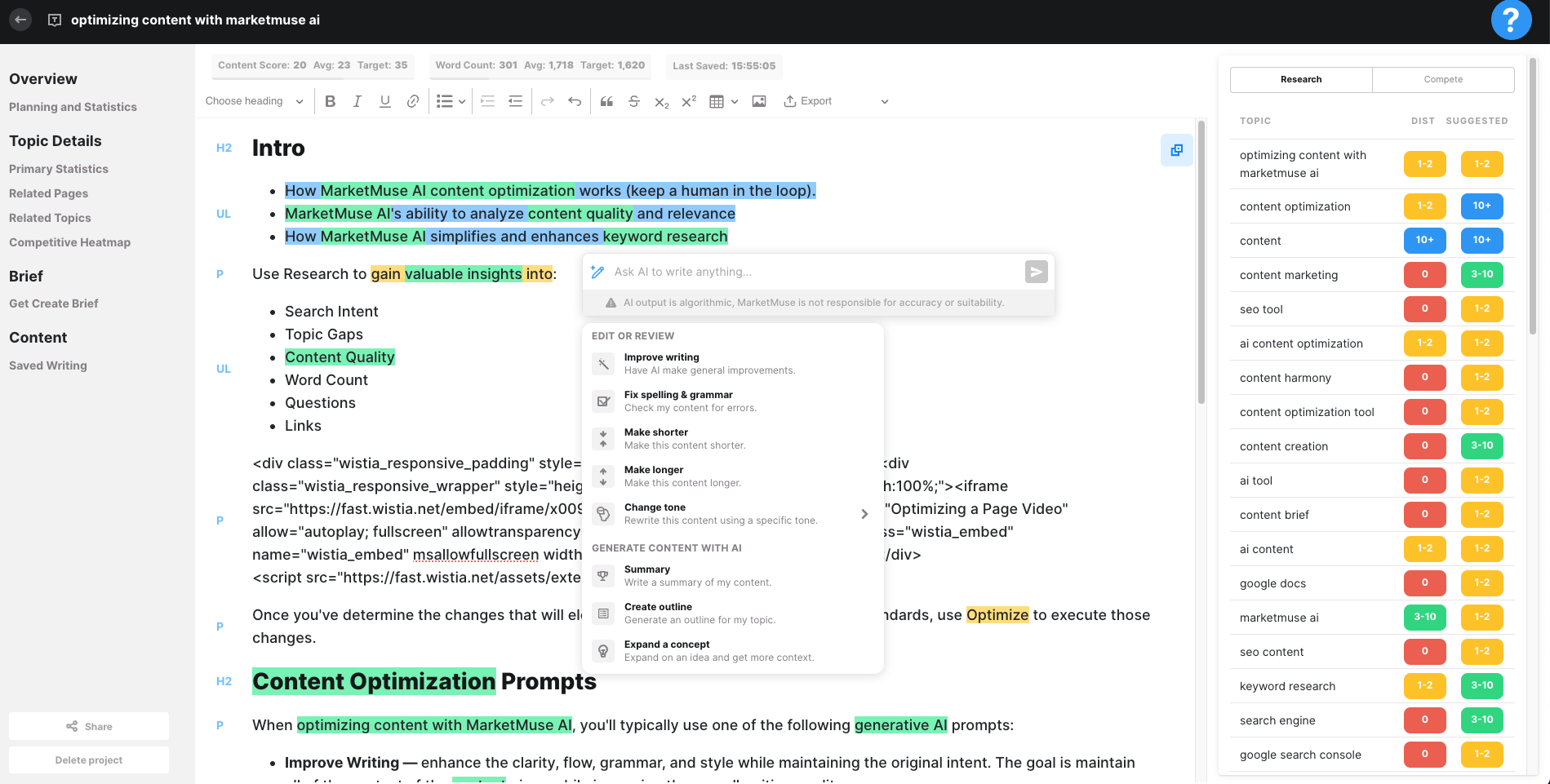
4. Monitor Quality Standards
Although large language models are trained on huge amounts of data, that doesn’t guarantee that the output:
- Is always accurate and impartial.
- Gives complete coverage.
- Corresponds to the reading grade level of your crowd.
- Is of a suitable word count.
By keeping an eye on these metrics, content creators can make sure that their content is of the best quality and accuracy, while helping to find possible areas for betterment.
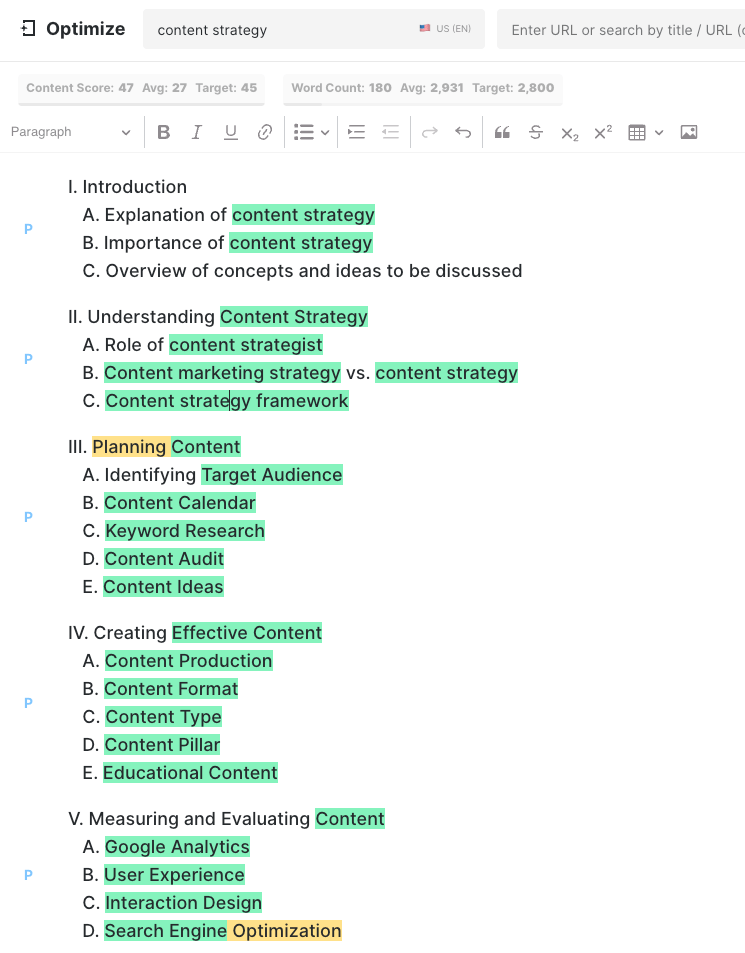
5. Supply Context and Directions
This step is essential in making sure that a large language model produces precise, dependable, and applicable output that aligns with your goals. It’s another reason you want to begin with a clear aim and intention. By expressing your desired objectives, details, or restrictions, you can guide the model towards providing more suitable content and limit unwanted behavior or biases. It also makes it easier to satisfy those quality metrics you’re tracking.
6. Gradually Refine the Output
This strategy helps improve accuracy and applicability, customize and optimize content, detect and fix mistakes, and give quality assurance and control. It also supports a continuous learning and progress attitude, allowing you to adjust to changing user requirements and preferences. This active engagement in the content creation process guarantees that the generated output meets your desired standards of accuracy and quality.
Again, this makes tracking those quality metrics a no-brainer. Plus, this approach fits well with a writer who’s interacting with the LLM, iterating on its output.
7. Verify and Cross-Check
Large language models never lie, but that doesn’t mean they tell the truth. Although they excel at mimicking human intelligence, they have no concept of truth or falsehood.
So verifying and checking content produced by language models is essential for accuracy, reliability, and trustworthiness. It also helps address biases, identify errors and gaps, encourage responsible content creation, and observe legal and ethical considerations. Plus, this strategy demonstrates professional integrity and builds trust with your audience.
Don’t land in hot water like this lawyer who used ChatGPT to self-verify the court citations it created. When asked, ChatGPT claimed that the citations it created were real, when in fact they weren’t. Alway use an external source, preferably a human, to validate any facts.
Takeaways
By following these steps, content creators can ensure that their output is of the highest quality and accuracy. Additionally, these strategies help address ethical considerations and build trust with the audience. With the right strategies in place, creating content with a large language model can be an effective and efficient way to generate high-quality content.
What you should do now
When you’re ready… here are 3 ways we can help you publish better content, faster:
- Book time with MarketMuse Schedule a live demo with one of our strategists to see how MarketMuse can help your team reach their content goals.
- If you’d like to learn how to create better content faster, visit our blog. It’s full of resources to help scale content.
- If you know another marketer who’d enjoy reading this page, share it with them via email, LinkedIn, Twitter, or Facebook.
Stephen leads the content strategy blog for MarketMuse, an AI-powered Content Intelligence and Strategy Platform. You can connect with him on social or his personal blog.