How to Use NLP in Content Marketing
Chris Penn, co-founder of Trust Insights, and MarketMuse Co-founder and Chief Product Officer Jeff Coyle discuss the business case for AI for marketing. After the webinar, Paul participated in an ask-me-anything session in our Slack Community, The Content Strategy Collective (join here). Here are the webinar notes followed by a transcript of the AMA.
The Webinar
The Problem
With the explosion in content we have new intermediaries. They’re not journalists or social media influencers. They are algorithms; machine learning models that dictate everything that stands between you and your audience.
Fail to account for this and your content will continue to be mired in obscurity.
The Solution: Natural Language Processing
NLP is the the programming of computers to process and analyze large amounts of natural language data. That comes from documents, chatbots, social media posts, pages on your website and anything else that’s essentially a pile of words. Rule-based NLP came first but was superseded by statistical natural language processing.
How NLP Works
The three core tasks of natural language processing are recognition, understanding, and generation.
Recognition – Computers can’t process text like humans. They can only read numbers. So the first step is converting the language into a format the computer can understand.
Understanding – Representing text as numbers enables algorithms to conduct statistical analysis to determine what topics are most frequently mentioned together.
Generation – After analysis and mathematical understanding, the next logical step in NLP is text generation. Machines can be used to surface the questions a writer needs to answer within their content. At another level, artificial intelligence can drive content briefs that provide additional insight into creating expert-level content.
These tools are commercially available today through MarketMuse. Beyond this are the natural language generation models that you can play with today, but aren’t in a commercially usable form. Although MarketMuse NLG Technology will be coming very soon.
Additional Resources Mentioned
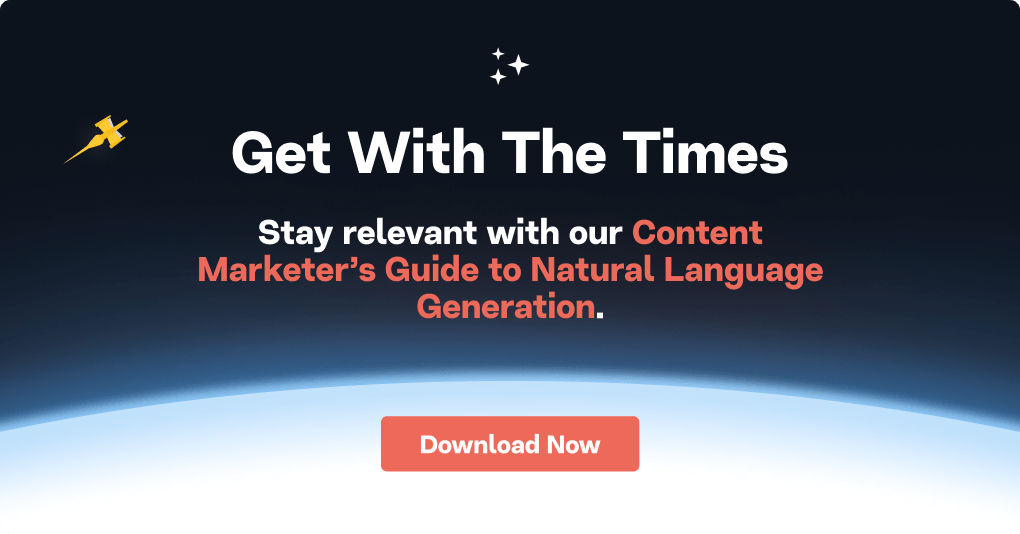
The AMA
Do you have any articles or website recommendations to keep up with AI industry trends?
Be reading the academic research published out there. Sites like these all do a great job of covering the latest and greatest.
That and major research publication hubs at Facebook, Google, IBM, Microsoft, and Amazon. You’ll see tons of great material shared on those sites.
“I’m using a keyword density checker for all of my content. How far removed from being a reasonable strategy is this today for SEO?”
Keyword density is essentially term frequency counting. It has its place for understanding the very rough nature of the text, but it lacks any kind of semantic knowledge. If you don’t have access to NLP tools, at least look at stuff like “people also searched for” content in the SEO tool of your choice.
Could you give some specific examples on how you generate content into… web pages? Posts? tweets?
The challenge is that these tools are exactly that – they’re tools. It’s like, how do you operationalize a spatula? It depends on what you’re cooking. You can use it to stir soup and also flip pancakes. The way to get started with some of this knowledge depends on your level of technical skill. If you’re comfortable with Python and Jupyter notebooks, for example, you can literally import the transformers library, feed in your training text file, and begin generation immediately. I did that with a certain politician’s tweets and it started spitting out tweets that would start World War 3. If you’re not technically comfortable, then start looking at tools like MarketMuse. I’ll let Jeff Coyle offer suggestions about how the average marketer gets started there.
If you look beyond tools, but more into strategies, what could be an example of a strategy that you could implement to make use of this knowledge?
A couple quick hit ones are for things like meta descriptions, for classifying pages or content blocks into a taxonomy, or for trying to guess questions that need answers — but those are really point solutions. The greater strategic wisdom comes when you use this to show you your current strengths, your gaps, and where you have momentum. From there, making decisions about what to create, update, expand becomes transformative for a business. Now imagine doing the same against a competitor. Finding their gaps. lather, rinse, repeat.
The strategy is always based on the goal. What goal are you trying to achieve? Are you attracting search traffic? Are you doing lead generation? Are you doing PR? NLP is a bunch of tools. It’s similar to – strategy is the menu. Are you serving breakfast, lunch, or dinner? What tools and recipes you use will be highly dependent on the menu you’re serving. A soup pot is going to be profoundly unhelpful if you’re making spanakopita.
What is a good starting place for someone who wants to begin mining data for insights?
Start with the scientific method.
- What question do you want to answer?
- What data, processes, and tools do you need to answer that question?
- Formulate a hypothesis, a single-condition, provably true or false statement you can test.
- Test.
- Analyze your test data.
- Refine or reject the hypothesis.
For the data itself, use our 6C data framework to judge the quality of the data.
What are, in your opinion, the main search user intents that marketers should take into consideration?
The steps along the customer journey. Map out the customer experience from beginning to end – awareness, consideration, engagement, purchase, ownership, loyalty, evangelism. Then map out what the intents are likely to be at each stage. For example, at ownership, the search intents are highly likely to be service-oriented. “How to fix airpods pro crackling noise” is an example. The challenge is collecting data at each of the stages of the journey and using that to train/tune.
Don’t you think this can be a bit volatile? If we need something more stable to automatize the process then we need to generalize things on a higher level.
Jeff Bezos famously said, focus on what doesn’t change. The general path to ownership doesn’t change much – someone unhappy with their pack of chewing gum will experience similar things as someone unhappy with the new nuclear aircraft carrier they commissioned. The details change, for sure, but understanding what types of data and intents is vital for knowing where someone is, emotionally, in a journey – and how they convey that in language.
What are the likely pitfalls people will fall in when trying to do user intent classification?
By far, confirmation bias. People will project their own assumptions onto the customer experience and interpret customer data through their own biases. I would also suggest to the extent possible you use interaction data (emails opened, feet in the door, calls to the call center, etc.) as best as you can to validate it. I know some places, especially larger organizations, are big fans of structured equation modeling to understand user intent. I wasn’t as much of a fan as they were, but it’s an additional potential approach.
What are tools or products that you think do a good job in determining the user intent of a query?
Woof. Besides MarketMuse? Honestly, I’ve had to work with my own stuff because I’ve not found great results, especially from mainstream SEO tools. FastText for vectorization and then unstructured clustering.
In your experience, how did BERT change Google Search?
BERT’s primary contribution is context, especially with modifiers. BERT allows Google to see word order and have it interpret meaning. Prior to that, these two queries might be functionally equivalent in a bag of words style model:
- where is the best coffee shop
- where is the best place to shop for coffee
Those two queries, while very similar, could have drastically different outcomes. A coffee shop might not be a place you want to buy beans. A Walmart is DEFINITELY not a place you want to drink coffee.
Do you think AI or ICT’s will ever develop consciousness/emotions/empathy like humans? How will we program them? How can we humanize AI?
The answer to that depends on what happens with quantum computing. Quantum allows for variable fuzzy states and massively parallel computing that mimics what’s happening in our own brains. Your brain is a very slow, chemical-based massive parallel processor. It’s really good at doing a bunch of things at once, if not quickly. Quantum would allow computers to do the same thing, but much, much faster – and that opens the door to artificial general intelligence. Here’s my concern, and this is a concern with AI today, already, in narrow usage: we train them based on us. Humanity has not done a great job of treating itself or the planet we live on well. We don’t want our computers to mimic that.
I suspect to the extent that systems permit, computer emotions will be functionally very different from our own and will self-organize from their data, just as ours do from our chemically-based neural networks. That in turn means they may feel very differently than we do. If machines, based principally in logic and data, do a candid, objective assessment of humanity, they may determine that frankly, we’re more trouble than we’re worth. And they wouldn’t be wrong, frankly. We are, as a species, a barbaric mess most of the time.
In your opinion, how do you see content marketers integrating/adopting Natural Language Generation into their daily workflow/processes?
Marketers should already be integrating some form of it, even if it’s just answering questions like we demoed in MarketMuse’s product. Answering questions that you know the audience cares about is a fast, easy way to create meaningful content. My friend Marcus Sheridan wrote a great book, “They Ask, You Answer” which ironically you don’t actually need to read in order to grasp the core customer strategy: answer people’s questions. If you don’t have questions submitted by real people yet, use NLG to make them.
Where do you see AI and NLP advancing in the next 2 years?
If I knew that, I would not be here, because I would be at the mountaintop fortress I purchased with my earnings. But in all seriousness, the major pivot we’ve seen in the last 2 years that shows no sign of change is the progression from “roll your own” models to “download pre-trained and fine-tune”. I think we’re due for some exciting times in video and audio as machines get better at synthesis. Music generation, in particular, is RIPE for automation; right now machines generate thoroughly mediocre music at best and ear-sores at worst. That is changing rapidly. I see more examples like blending transformers and autoencoders together like BART did as major next steps in model progression and state of the art results.
Where do you see Google research heading with regard to informational retrieval?
The challenge Google continues to face, and you see it in many of their research papers, is scale. They’re especially challenged with stuff like YouTube; the fact that they still rely heavily on bigrams isn’t a knock on their sophistication, it’s an acknowledgment that anything more than that has an insane computational cost. Any major breakthroughs from them aren’t going to be at the model level so much as at the scale level to deal with the deluge of new, rich content being poured onto the internet every day.
What are some of the most interesting applications of AI you’ve come across?
Autonomous everything is an area I watch closely. So are deep fakes. They are examples of just how perilous the road ahead is, if we’re not careful. In NLP specifically, generation is making rapid strides and is the area to watch.
Where have you seen SEOs use NLP in ways that don’t work or won’t work?
I’ve lost count. A lot of the time, it’s people using a tool in a way that it wasn’t intended and getting subpar results. Like we mentioned on the webinar, there are scorecards for the different state of the art tests for models, and people who use a tool in an area it’s not strong don’t typically enjoy the results. That said… most SEO practitioners aren’t using any kind of NLP aside from what vendors provide them, and many vendors are still stuck in 2015. It’s all keyword lists, all the time.
Where do you see video (YouTube) and Image search at Google? Do you think the technologies deployed by Google used for all types of searches are very similar or different from each other?
Google’s technologies are all built on top of their infrastructure and use their tech. So much is built on TensorFlow and for good reason – it’s super robust and scalable. Where things vary is in how Google uses the different tools. TensorFlow for image recognition inherently has very different inputs and layers than TensorFlow for pairwise comparison and language processing. But if you know how to use TensorFlow and the various models out there, you can achieve some pretty cool stuff on your own.
In what ways can we adapt/keep up with advancements in AI and NLP?
Keep on reading, researching, and testing. There’s no substitute for getting your hands dirty, at least a little. Sign up for a free Google Colab account and try things out. Teach yourself a little Python. Copy and paste code examples from Stack Overflow. You don’t need to know every inner working of an internal combustion engine to drive a car, but when something goes wrong, a little knowledge goes a long way. The same is true in AI and NLP – even just being able to call BS on a vendor is a valuable skill. It’s one of the reasons I enjoy working with the MarketMuse folks. They actually know what they’re doing and their AI work isn’t BS.
What would you say to people who are worried about AI taking their jobs? For example, writers who see technology like NLG and worry they’ll be out of work if the AI can be “good enough” for an editor to just clean the text up a bit.
“AI will replace tasks, not jobs” – the Brookings InstituteAnd it’s absolutely true. But there will be net jobs lost, because here’s what will happen. Suppose your job is composed of 50 tasks. AI does 30 of them. Great, you now have 20 tasks. If you’re the only person that does that, then you’re in nirvana because you have 30 more units of time to do more interesting, more fun work. That’s what the AI optimists promise.Reality check: if there are 5 people doing those 50 units, and AI does 30 of then, then AI is now doing 150 / 250 units of work. That means that there are 100 units of work left for people to do, and corporations being what they are, they will immediately cut 3 positions because the 100 units of work can be done by 2 people.Should you be worried about AI taking jobs? It depends on the job. If the work you do is incredibly repetitive, absolutely be worried. At my old agency, there was a poor sod whose job it was to copy and paste search results into a spreadsheet for clients (I worked at a PR firm, not the most technologically advanced place) 8 hours a day. That job is in immediate danger, and frankly should have been for years.Repetition = automation = AI = task loss. The less repetitive your work, the safer you are.
Each change also created more and more income inequality. We are now at a dangerous point where machines – which do not spend, are not consumers – are doing more and more work of people who do spend, who do consume, and we see this in the massive wealth dominance in technology. That’s a societal issue we’re going to have to tackle at some point.
And the challenge with that is progress is power. As Robert Ingersoll wrote (and was later misattributed to Abraham Lincoln): ““Nearly all men can stand adversity, but if you want to test a man’s character, give him power.”We see how people today are handling power.
How can I pair Google Analytics data with NLP Research?
GA indicates direction, then NLP indicates creation. What’s popular? I just did this for a client a little while ago. They have thousands of web pages and chat sessions. We used GA to analyze which categories were growing fastest on their site and then used NLP to process those chat logs to show them what’s trending and what they needed to create content about.
Google Analytics is great for telling us WHAT happened. NLP can start to tease out a little bit of the WHY, and then we complete that with market research.
I’ve seen you use Talkwalker as a data source in many of your studies. What other sources and use cases should I consider for analysis?
So, so many. Data.gov. Talkwalker. MarketMuse. Otter.ai for transcribing your audio. Kaggle kernels. Google Data Search – which by the way is GOLD and if you don’t use it, you absolutely should be. Google News and GDELT. There are so many great sources out there.
What does an ideal collaboration between marketing and data analytics team look like to you?
Not joking; one of the biggest mistakes Katie Robbert and I see all the time at clients are organizational silos. The left hand has no idea what the right hand is doing, and it’s a hot mess everywhere. Getting people together, sharing ideas, sharing to-do lists, having common standups, teaching each other – functionally being “one team, one dream” is the ideal collaboration, to the point where you don’t need to use the word collaboration anymore. People just work together and bring all their skills to the table.
Can you review the MVP report that you frequently preview in your presentations and how it works?
The MVP report stands for most valuable pages. The way it works is by extracting path data from Google Analytics, sequencing it, and then putting it through a Markov chain model to ascertain which pages are most likely to assist conversions.
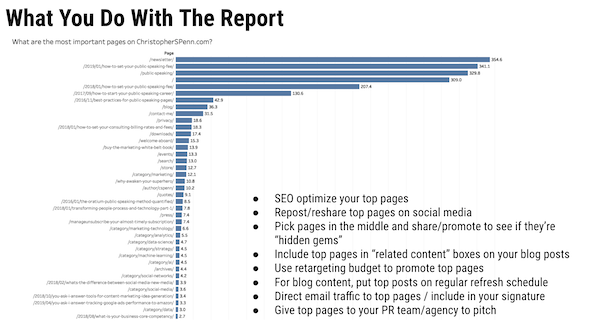
And if you want the longer explanation.
Can you give some more insight into data bias? What are some considerations when building NLP or NLG models?
Oh yes. There’s so much to say here. First, we need to establish what bias is, because there are two fundamental kinds.
Human bias is generally accepted to be defined as “Prejudice in favor of or against something compared to another, usually in a way considered to be unfair.”
Then there’s mathematical bias, generally accepted to be defined as “A statistic is biased if it is calculated in such a way that it is systematically different from the population parameter being estimated.”
They are different but related. Mathematical bias isn’t necessarily bad; for example, you absolutely want to be biased in favor of your most loyal customers if you have any business sense whatsoever. Human bias is implicitly bad in the sense of unfairness, especially against anything that’s considered to be a protected class: age, gender, sexual orientation, gender identity, race/ethnicity, veteran status, disability, etc. These are classes that you MUST NOT discriminate against.
Human bias begets data bias, typically in 6 places: people, strategy, data, algorithms, models, and actions. We hire biased people – just look at the executive suite or board of directors of a company to determine what its bias is. I saw a PR agency the other day touting its commitment to diversity and one click to their executive team and they’re a single ethnicity, all 15 of them.
I could go on for QUITE some time about this but I’ll suggest that you take a course I developed on this topic, over at the Marketing AI Institute. In terms of NLG and NLP models, we have to do a few things.
First, we have to validate our data. Is there a bias in it, and if so, is it discriminatory against a protected class? Second, if it is discriminatory, is it possible to mitigate against it, or do we have to throw the data out?
A common tactic is to flip metadata to debias. If you have, for example, a dataset that is 60% male and 40% female, you recode 10% of the males to female to balance it for model training. That’s imperfect and has some issues, but it’s better than letting the bias ride.
Ideally, we built interpretability in our models that allow us to run checks during the process, and then we also validate the results (explainability) post hoc. Both are necessary if you want to be able to pass an audit certifying you’re not building biases into your models. Woe is the company that only has post hoc explanations.
And finally, you absolutely need human oversight of a diverse and inclusive team to verify the outcomes. Ideally you use a third party, but a trusted internal party is okay. Does the model and its outcomes present a skewed result than you would get from the population itself?
For example, if you were creating content for 16-22 year olds and you didn’t once see terms like deadass, dank, low-key, etc. in the generated text, you’ve failed to capture any data on the input side that would train the model to use their language accurately.
The biggest main challenge here is dealing with all that through unstructured data. That’s the reason lineage is SO important. Without lineage, you can’t prove that you sampled the population correctly. Lineage is your documentation of what the data source is, where it came from, how it was collected, whether any regulatory requirements or disclosures apply to it.
What you should do now
When you’re ready… here are 3 ways we can help you publish better content, faster:
- Book time with MarketMuse Schedule a live demo with one of our strategists to see how MarketMuse can help your team reach their content goals.
- If you’d like to learn how to create better content faster, visit our blog. It’s full of resources to help scale content.
- If you know another marketer who’d enjoy reading this page, share it with them via email, LinkedIn, Twitter, or Facebook.
Stephen leads the content strategy blog for MarketMuse, an AI-powered Content Intelligence and Strategy Platform. You can connect with him on social or his personal blog.